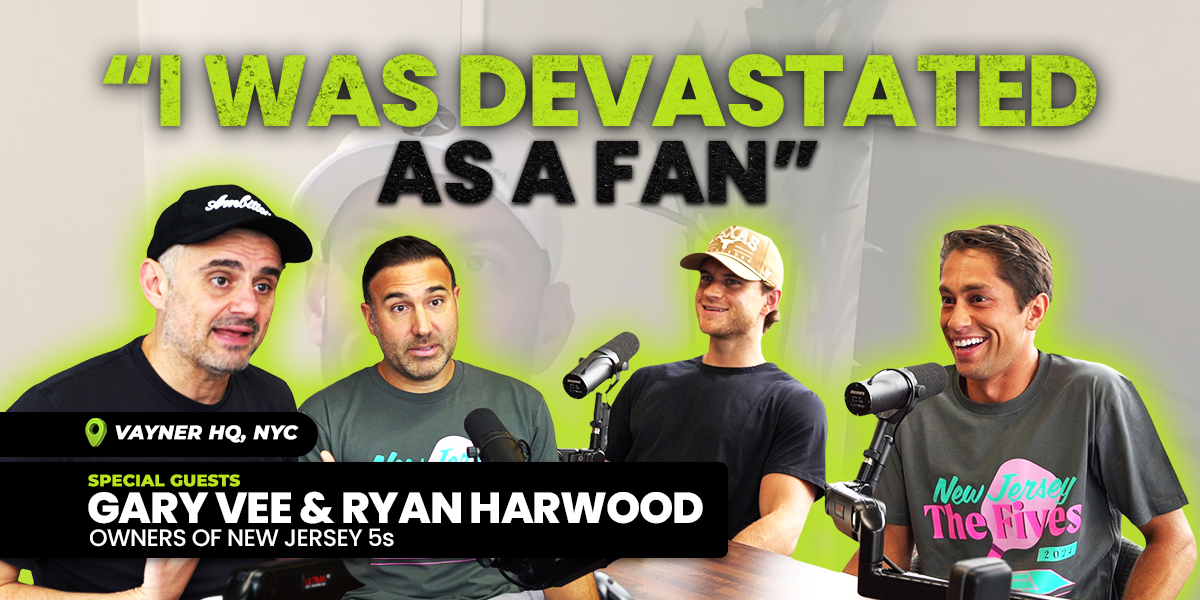
PicklePod: Gary Vee - Pickleball is the Future, Building the Next NBA
Gary Vaynerchuk, better known as Gary Vee, is a serial entrepreneur, bestselling author, and digital marketing pioneer who has become a leading voice in the world of business and social media. In addition to his many ventures, Gary is also an avid pickleball enthusiast and co-owner of the New Jersey 5s, a Major League Pickleball team.
His involvement in the sport has been pivotal in bringing more attention to pickleball's rapid rise. He combines his marketing expertise with a deep passion for growing the game.
In this episode of the PicklePod (subscribe here), Gary and New Jersey 5s GM Ryan Harwood discuss why pickleball is the future and provide insight into how they both got involved in MLP.
The Value of a Signed 5s Paddle
The episode starts with a lighthearted discussion about the rising value of pickleball memorabilia. Gary Vee humorously reflects on the potential future value of a signed paddle from his Major League Pickleball team, the New Jersey 5s:
“One day I expect to sell that paddle for $5,000.”
Gary then shares the origin story of how he and his team decided to invest in Major League Pickleball.
“We did probably the least amount of due diligence in history, but we knew this sport was about to blow up.”
Is This Your Fastest Return on Investment?
When asked about the speed of MLP's growth, Gary discusses how pickleball team valuations have skyrocketed, noting how the New Jersey 5s' worth has grown exponentially. While excited by the rapid growth, Gary remains cautious:
"It’s on paper until it’s real.”
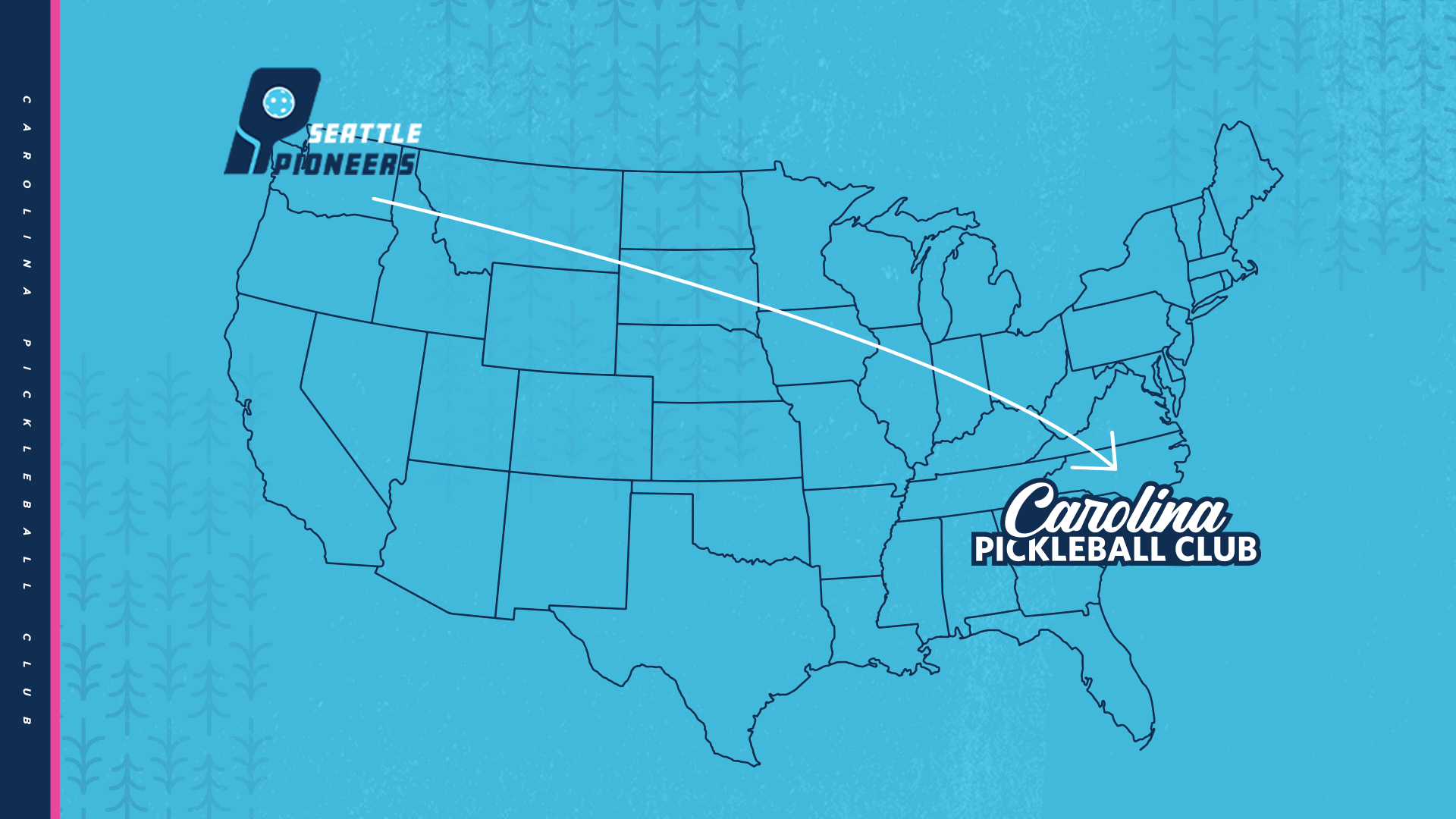
Pickleball Closes the Age and Gender Gap
One of the best moments in the podcast was the acknowledgment of pickleball’s ability to level the playing field for people of all ages and genders.
Gary tells a story of how most of us were introduced into pickleball.
“A 71-year-old grandma can whip the physical ass off her 23-year-old grandson.”
He states this accessibility makes pickleball unique and appealing to a broad audience.
From on Paper to Actual Cash Flow
Gary and the team discuss turning MLP from a rapidly appreciating asset into a sustainable, cash-flowing business. Sponsorship deals, media rights, and ticket sales are key factors in making this transition happen.
“The big elephant in the room is the media rights deal.”
14:36 MLP’s Lax Rules on Celebrations
One unique aspect of MLP that Gary appreciates is the openness to player celebrations, something that's not as accepted in more traditional sports.
“In the NFL, everyone celebrates after every play. Pickleball needs more of that raw emotion.”
He notes fan engagement and excitement come from these moments of passion on the court.
It's something the 5s fans always show up for whenever the team plays. This past weekend, they were especially vocal (usually at Gary's urging) in New York.
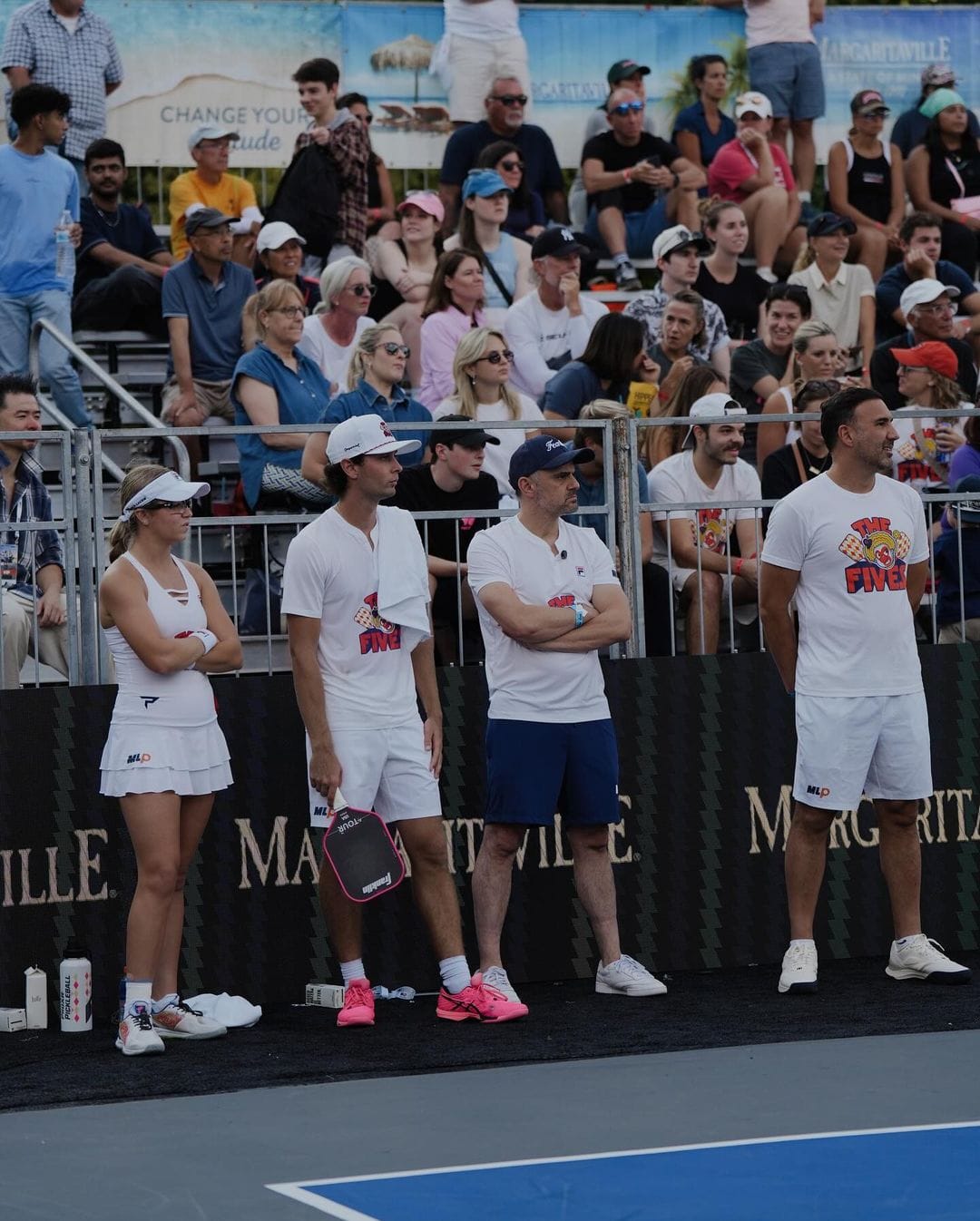
Gary Was Pot Committed to Anna Leigh Waters
One of New Jersey 5s' biggest moves was locking in Anna Leigh Waters, the sport's top female athlete.
Gary reveals his strategy for securing her, describing it as his “fantasy baseball trick” and emphasizing how important it was to the future of the team and the league.
MLP Won’t Reach Full Potential Until It Is City-Based
For MLP to reach its full potential, Gary believes it needs to adopt a city-based model, similar to the NBA or NFL. He argues that having teams tied to specific locations will build stronger fan loyalty:
“Team sports are epic because people fall in love with the team, not just the players.”
Building the Personal Brand as a Player
Gary emphasizes the importance of players building their personal brands beyond the court.
“There’s too much content that’s the same—players need to show who they are as people, not just how they dink.”
He encourages players to document their lives, not just create highlight reels, to connect with fans on a deeper level.
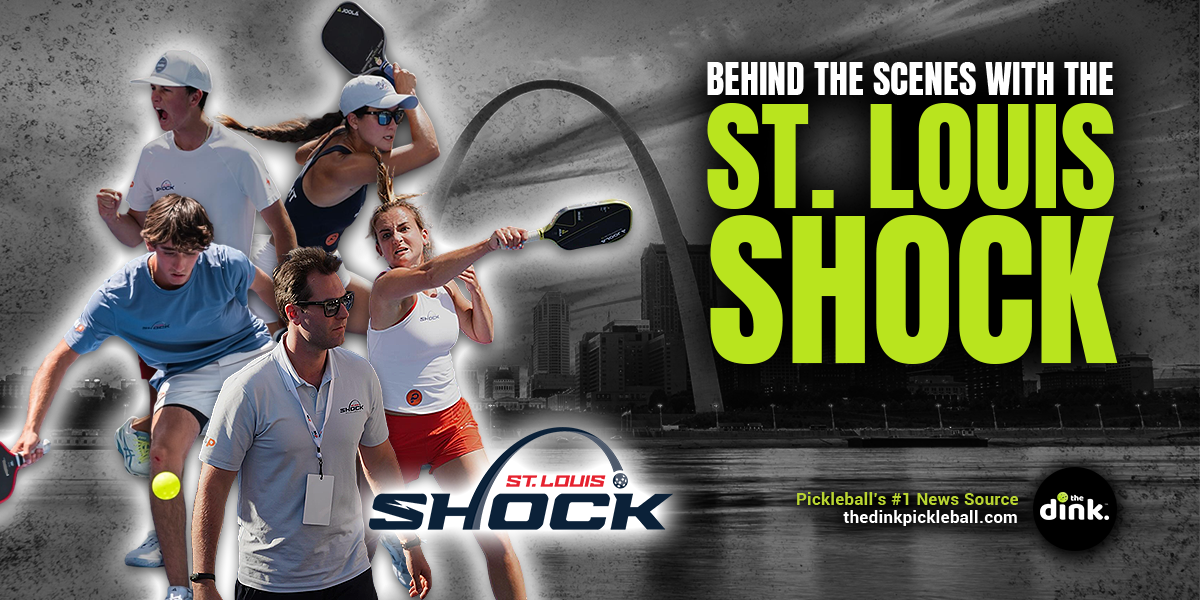
Overlooked Business Opportunities
While pickleball is booming, Gary admits there are still untapped business opportunities in the sport. From paddle companies to Topgolf-style pickleball facilities, there’s no shortage of areas to innovate.
For pickleball to go truly mainstream, Gary believes the sport needs to simplify how fans can watch it.
“We’ve got to remove the friction to watch it—make it easy, like turning on CBS for a football game.”
He argues that a unified media deal could be the key to taking the sport to the next level.
ATP Players Looking to Make the Switch to Pickleball
The episode touches on a growing trend: ATP tennis players considering switching to pickleball. Gary shares how many tennis pros have reached out to him for advice on making the transition, underscoring how appealing pickleball is becoming as a career option.
“Yeah, you should take six months and go all in.”
What’s the Pitch to Potential Brands?
Gary wraps up by sharing how he pitches potential sponsors for the New Jersey 5s. It’s all about the combination of “social media content, events, and branding.” The growing viewership and buzz around the sport make it an exciting opportunity for brands to align with pickleball's rapid rise.
|