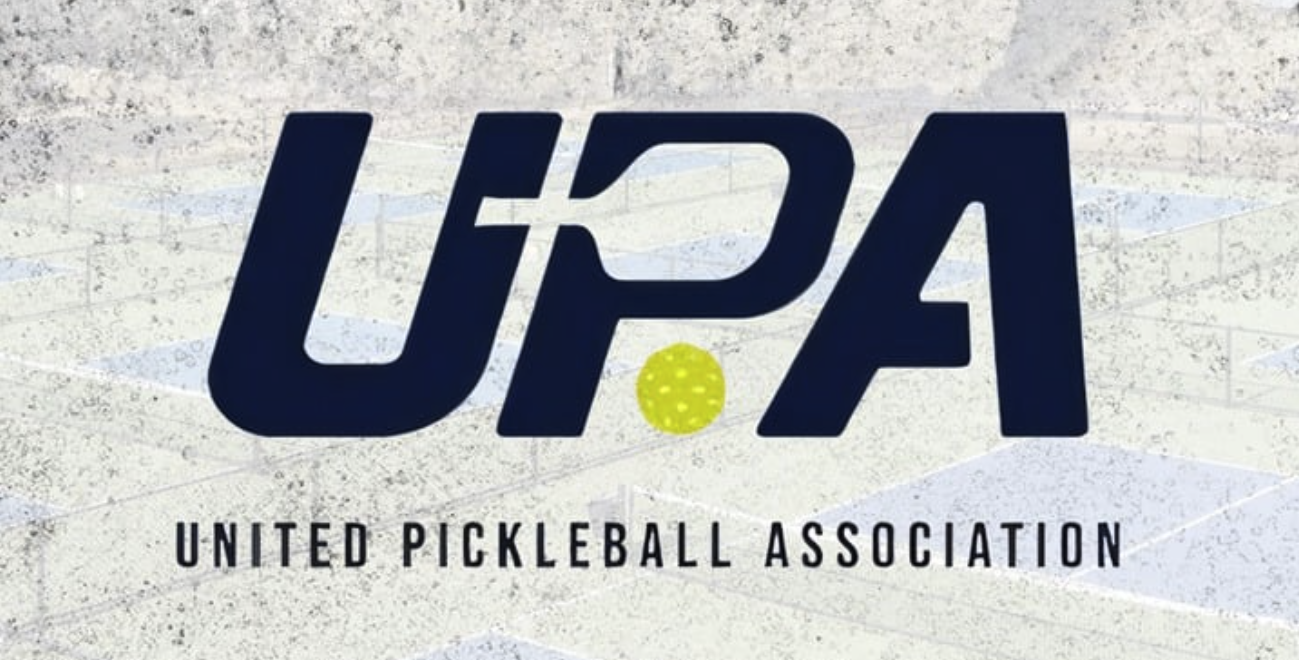
The United Pickleball Association of America (UPA-A) today announced an amendment to it "reckless targeting" rule, first rolled out just a few weeks ago. It's now referred to as "head hunting" – and it will no longer be called by match officials during live game play.
The "Updated Provisional Rule: Head Hunting," published to the UPA-A site today, places the same emphasis on "maintaining the integrity of the game while prioritizing player safety" by penalizing players who intentionally target an opponent above the shoulders.
On paper, it's a new name for the same offense — "reckless targeting above the shoulders" is really just a longer way of saying "head hunting."
But there are some key differences in how an infraction will be interpreted and penalized.
What's New?
The difference with the new "head hunting" rule is how it will be enforced: Whereas before, officials were expected to analyze the intent of a player and make such a call in real time, now that pressure is off.
"If a player deliberately or recklessly targets an opponent with a powerful shot
at an opponent’s head," they said, "the point will be reviewed by the UPA-A post match."
Meaning, intent will be determined by video review, not an official's snap judgement.
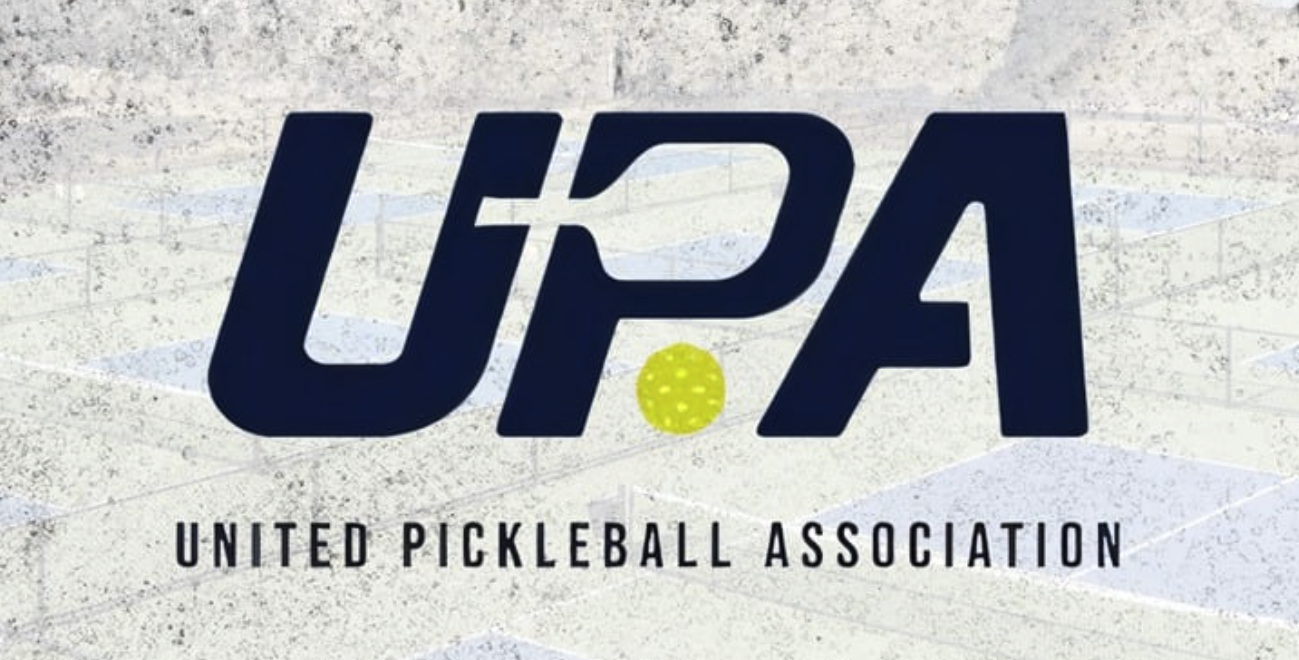
Increased Fines
If Head Hunting is determined, the offending player will receive the following:
First Offense in a 3 month period — There will be a minimum fine of $5,000 to the offending player.
Second Time Offense in a 3 month period — there will be a minimum fine of $10,000 and the player may be subject to suspension.
The minimum, first-offense fine has been increased from $2,500 to $5,000. The updated rule also introduces a three-month window for first and second offenses.
Additionally, "repeat offenders or particularly egregious instances," they add, "may result in additional penalties, fines, or suspensions at the discretion of tournament officials and the UPA-A disciplinary committee."
Rationale and Enforcement
This remains unchanged from the previous "reckless targeting" rule.
While the modern game has become faster and more aggressive, respect for opponents remains paramount. Players are encouraged to compete with intensity but must avoid endangering fellow competitors. UPA-A officials will assess intent, ball trajectory, and overall gameplay context when determining violations.
This rule is designed to preserve both player safety and the integrity of professional competition, ensuring that pickleball remains a sport built on skill, strategy, and sportsmanship.
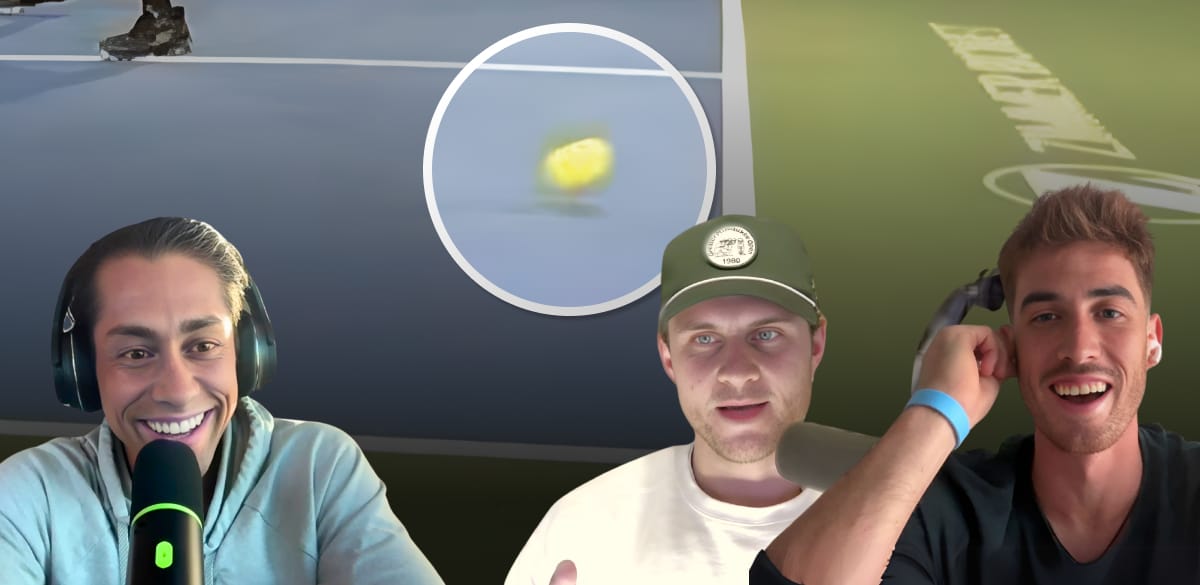
Why This Matters
The only instance of "reckless targeting" to-date came in a match involving Hayden Patriquin, who sent a high heater toward Wyatt Stone.
(p.s if you're curious, check out the paddle Hayden was using for this match)
It ended up hitting him in the arm, but match official Don Stanley signaled a "reckless targeting" violation before quickly walking it back.
These types of plays are going to happen, whether intentionally or not, and pros seem split on whether they should come with a fine or not.
Federico Staksrud talked about this on the latest episode of the PicklePod.
"I think that rule is dumb, if you can hit someone in the face, and you want to do it, you should be able to," he said, quickly clarify that he would never hit such a shot intentionally.
I am going to leave this link to Amazon's best selling pickleball eyewear here just in case.